The effects of the COVID-19 pandemic on our healthcare system are well-documented and ongoing, as are the effects on our economy. While lockdowns have fortunately subsided, the need for those annoying nasopharyngeal PCR and RAT tests persists. According to a recent paper in Nature, a team of researchers have devised a game-changing non-invasive, microscopy-based technique that has demonstrated a very high success rate for detecting the SARS-CoV-2 virus in preclinical testing.
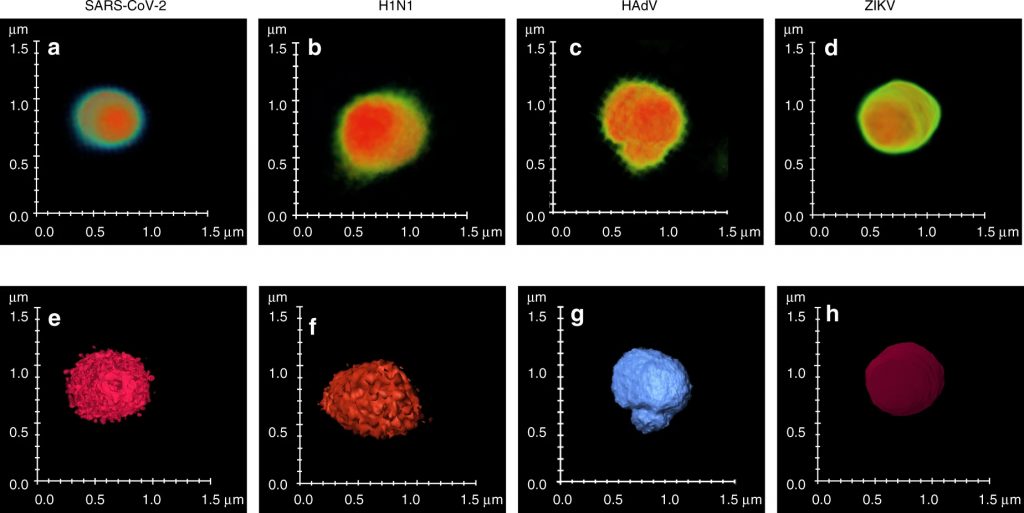
The study carried out by researchers from the Beckman Institute, the University of Illinois Urbana-Champaign, and the University of Illinois at Chicago lead by Professor Gabriel Popescu have devised an innovative label-free approach, using a technique called Spatial Light Interference Microscopy (SLIM) previously developed at the Beckman Institute and applying artificial intelligence (AI).
Most viruses are difficult to image by light microscopy because their size is below the diffraction limit of light and hence electron microscopy (EM) is normally the preferred method for imaging. EM unfortunately requires extensive sample preparation. When asked how SLIM, a light microscopy technique could be used, Neha Goswami, a graduate student in bioengineering said, “Applying SLIM for virus imaging is like looking at something without your glasses on. The image is blurry due to the viruses being smaller than the diffraction limit. However, owing to the high sensitivity of SLIM, we can not only detect the viruses, but also differentiate between different types.”
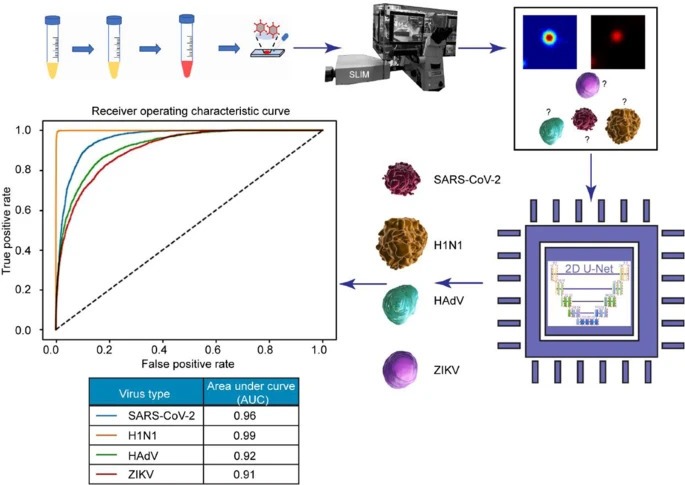
Using the SLIM data with some specific training to program a neural network the researchers were able to differentiate between a number of viruses. They used fluorescence imaging for confirmation and training so that the AI could soon accurately detect the virus subsequently using just the SLIM image.
To really test the system, they introduced other viruses including H1N1, Human Adenovirus and Zika virus as well as dust. Under these conditions, the system was able to accurately detect the SARS-CoV-2 virus 96% of the time in less than a minute on a glass slide, making it an extremely fast technique.
The technique is undergoing clinical trials, including a non-invasive sample breath testing procedure. The ultimate goal is to develop a sensitive and specific test that is also fast, affordable and scalable, with the potential for portability and point-of-care applications. Commenting on the current state of play, Prof. Popescu commented, “Our current efforts are focused on demonstrating this approach in the clinic and deploying it worldwide for COVID and potentially other infectious diseases”.
While currently being developed to combat COVID-19, this highly adaptive AI programming could be used in future pandemics and other scenarios as a fast technique for disease detection.
Read the full paper at https://www.nature.com/articles/s41377-021-00620-8
Goswami, N., He, Y.R., Deng, YH. et al. Label-free SARS-CoV-2 detection and classification using phase imaging with computational specificity. Light Sci Appl 10, 176 (2021).